Eruption forerunners from multiparameter monitoring and application for eruptions time predictability (Piton de la Fournaise)
Abstract
Volcanic eruptions impact on societal risk, and volcanic hazard assessment is a necessary ingredient for decision‐makers. However, the prediction of volcanic eruptions remains challenging due to the complexity and the non‐linearity of volcanic processes. Identified forerunners such as increasing seismicity or deformation of the volcanic edifice prior to eruption are not deterministic. In this study, we use statistical methods to identify and discriminate precursory patterns to eruptions, on three sets of observables of Piton de la Fournaise volcano. We analyzed the short‐term (i.e. the inter‐eruptive period) time series of the seismicity rate, the deformation and the seismic velocity changes (deduced from seismic noise cross‐correlations) over the period 1999–2006, with two main goals. First, we characterize the average pre‐eruptive time patterns before 22 eruptions using superposed epoch analysis for the three observables. Using daily rate values, we resolve (1) a velocity change within 100–50 days from the eruptions onsets, then a plateau value up to eruption onset; (2) a power law increase in seismicity rate from noise level 15–10 days before eruption time; (3) an increase of displacement rate on the eruption day. These results support a three step mechanism leading to magma transfers toward the surface. Second we use pattern recognition techniques and the formalization of error diagrams to quantify the predictive power of each forerunner either as used independently or as combined to each other. We show that when seismicity rate alone performs the best prediction in the failure to predict versus alarm duration space, the combination of the displacement and seismicity data reduces the false alarm rate. We further propose a tool which explores the prediction results in order to optimize prediction strategy for decision‐makers, as a function of the risk value.
full article: https://agupubs.onlinelibrary.wiley.com/doi/full/10.1029/2012JB009167
Volcanic eruptions impact on societal risk, and volcanic hazard assessment is a necessary ingredient for decision‐makers. However, the prediction of volcanic eruptions remains challenging due to the complexity and the non‐linearity of volcanic processes. Identified forerunners such as increasing seismicity or deformation of the volcanic edifice prior to eruption are not deterministic. In this study, we use statistical methods to identify and discriminate precursory patterns to eruptions, on three sets of observables of Piton de la Fournaise volcano. We analyzed the short‐term (i.e. the inter‐eruptive period) time series of the seismicity rate, the deformation and the seismic velocity changes (deduced from seismic noise cross‐correlations) over the period 1999–2006, with two main goals. First, we characterize the average pre‐eruptive time patterns before 22 eruptions using superposed epoch analysis for the three observables. Using daily rate values, we resolve (1) a velocity change within 100–50 days from the eruptions onsets, then a plateau value up to eruption onset; (2) a power law increase in seismicity rate from noise level 15–10 days before eruption time; (3) an increase of displacement rate on the eruption day. These results support a three step mechanism leading to magma transfers toward the surface. Second we use pattern recognition techniques and the formalization of error diagrams to quantify the predictive power of each forerunner either as used independently or as combined to each other. We show that when seismicity rate alone performs the best prediction in the failure to predict versus alarm duration space, the combination of the displacement and seismicity data reduces the false alarm rate. We further propose a tool which explores the prediction results in order to optimize prediction strategy for decision‐makers, as a function of the risk value.
full article: https://agupubs.onlinelibrary.wiley.com/doi/full/10.1029/2012JB009167
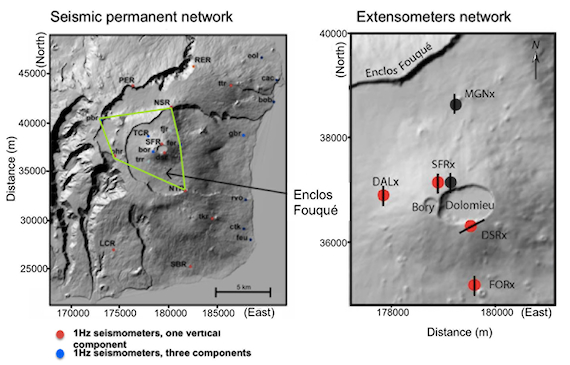
Commenti